Retinal Nerve Fiber Layer Optical Texture Analysis
All content on Eyewiki is protected by copyright law and the Terms of Service. This content may not be reproduced, copied, or put into any artificial intelligence program, including large language and generative AI models, without permission from the Academy.
Overview
The current standard to detect structural damage in glaucoma patients is based on examining the retinal nerve fiber layer (RNFL). Several techniques, such as optical coherence tomography (OCT) and red-free photography, are currently used to detect RNFL defects. [1] Oddone et al. conducted a systematic review including 34 studies (5226 eyes). They reported that the average RNFL thickness sensitivity using RTVue OCT was 0.66, and the average specificity was 0.95. They also found that the relative diagnostic odds ratio (DOR) for RFNL thickness in glaucoma detection using conventional OCT was 34.06 (22.14–52.39) at a p-value ≤ 0.01.[2] Recently, Leung et al. have developed a new parameter known as RNFL Optical Texture Analysis (ROTA), which may be more accurate in detecting these RNFL defects.
How does ROTA’s algorithm work?
ROTA’s algorithm gathers RNFL thickness and RNFL reflectance measurements from a standard OCT scan. The steps in generating the ROTA algorithm are outlined below:
1. OCT is used to obtain raster retina scans covering the peripapillary region and macula.
2. The boundaries of the retinal nerve fiber layer are segmented.
3. The optical texture signature (Sxy) is computed using RNFL reflectance and thickness measurements taken from the OCT.
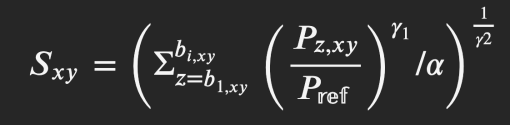
This formula was developed and described in detail by Leung et al. in order to determine the optical texture signature. Pz,xy, obtained from the OCT, represents optical density measurements at retinal locations (x, y) and depths (z). Sxy is computed using the optical density measurements at retinal locations between the anterior and posterior boundaries of the RNFL.[3]
● b1,xy and bi,xy correspond to anterior and posterior boundaries, respectively, of the RNFL at retinal location (x, y)
● Pref represents a reference optical density value for normalization
● γ1 represents a gamma value for a gamma transformation function
● γ2 represents a gamma value for a gamma correction function
● α represents a preset constant proportional to the peak tissue thickness of the RNFL. [3]
4. The optical texture signature value is used to generate a texture analysis map, and a machine learning algorithm is trained to detect abnormalities in the RNFL based on this map.
ROTA’s advantage comes from its ability to reveal wide areas of the retina, including the trajectories and optical textures of the arcuate bundles, papillomacular bundles, and nasal radial bundles. Additionally, as retinal vessels and axonal fiber bundles hold unique optical-texture signatures, the ROTA algorithm can distinguish between retinal vessels and the RNFL. [3] Current standards to detect glaucoma are compromised when non-glaucomatous optic neuropathies and other eye conditions coexist, such as myopia and ocular hypertension. [4] ROTA can detect these non-glaucomatous conditions and potentially differentiate them from glaucomatous optic neuropathies. This is because ROTAS’s technique provides comprehensive trajectories and optical textures over a wider field that may be used to detect distinctive loss patterns associated with specific optic neuropathies.[3]
The appeal of ROTA comes not only from its ability to improve diagnostic evaluations of glaucomatous and non-glaucomatous optic neuropathies but also from its feasibility. Because ROTA’s algorithm is generated using measurements obtained from commercially available OCT instruments, it can be readily applied to clinical settings that already use OCT instruments.[3]

ROTA compared to the current standards in the detection of glaucoma
Studies are ongoing to evaluate the effectiveness of ROTA in comparison to other parameters in the diagnostic evaluation of glaucoma. In locations where the RNFL is thin, ROTA can distinctly recognize RNFL defects in both early-stage and late-stage glaucoma (Figure 1) that are missed by red-free RNFL photography. This is because ROTA provides more comprehensive detail on axonal fiber bundles' optical texture and trajectories. [3] In their study on ROTA, Leung et al. examined 531 eyes of 363 patients with RNFL defects and 315 eyes of 177 healthy participants. Their examination included a diagnostic evaluation for glaucoma using ROTA, Cirrus HD-OCT, and red-free photography. The study reported that ROTA’s algorithm in detecting glaucoma (with red-free photography as a reference standard) can reach a sensitivity of 98.9% (95% CI: 95.4–100.0%, p-value = 0.002). The sensitivity of red-free photography in the detection of glaucoma in this study was lower, at 79.3% (95% CI: 66.3–91.0%), with a p-value of 0.002. [3]
In addition, because ROTA's algorithm corrects for axial length, it is less likely to lead to false-positive detection of RNFL/ganglion cell layer-inner plexiform layer (GC-IPL) abnormalities. In the same study by Leung et al., they reported that the specificity of ROTA was 94.3% (95% CI: 91.3–97.2%), which was higher than the specificity of OCT in detecting RNFL defects over the parapapillary region (pRNFLT) and GC-IPL region (87.9%; 95% CI: 83.6–92.3%) and (78.1%; 95% CI: 72.4–83.8%), respectively.
ROTA has also been used to reveal distinct axonal fiber bundle loss. Another study by Leung et al. showed that nerve damage in early glaucoma involves the papillofoveal and papillomacular bundles, which are harder to visualize using current standards. This study examined two hundred-four eyes (171 patients) with early glaucoma (visual field sensitivity mean deviation of at least –6 dB) using ROTA. They reported that 71.6% had RNFL defects involving the papillomacular bundle and 17.2% had RNFL defects involving the papillofoveal bundles. These RNFL defects were found to correlate to the visual field sensitivity of at least 1 of the four central visual field locations on pattern plots (P<0.05 in the pattern deviation probability plot; odds ratio, 12.5). However, this correlation was not seen for visual field depression in ≥3 non-edge contiguous locations.[6] Their results showed that RNFL defects are not limited to the hemiretina, as the fovea and macula were also involved. ROTA's ability to reveal this glaucomatous nerve damage in wider areas of the retina may give researchers a more accurate diagnostic tool for evaluating glaucoma, especially at early stages.
In patients with ocular hypertension, OCT can miss the detection of RNFL defects and is more likely to be detected by ROTA. A study conducted by Su et al. examined 600 eyes (306 patients) (mean age of 58.2±12.9 years) with ocular hypertension. They evaluated them by clinically examining the optic disc, doing OCT analysis, and ROTA. Of these eyes, none showed RNFL defects through clinical examination of the optic disc/RNFL or OCT analysis. However, ROTA analysis revealed that 10.8% of the patients had RNFL defects suggesting that the RNFL bundle defects detected by the ROTA may be the earliest sign of glaucoma development. The most common location of the RNFL defects was the superior arcuate bundle. Older age and higher pattern standard deviation were significantly associated with RNFL defects on ROTA. [4]
Using ROTA to discern glaucoma from non-glaucomatous optic neuropathies
Given the fact that ROTA can scan wide areas, including the arcuate, papillomacular, and nasal radial bundles, it can be used to discern specific forms of optic neuropathies and distinguish glaucoma from non-glaucomatous optic neuropathies.. [3] In the study on ROTA by Leung et al.[3], they examined and imaged patients with rim pallor. Thye reported that ROTA showed RNFL bundle loss corresponding to the area of the rim pallor. Such correlation may be difficult to be detected by the conventional OCT analysis. This preciseness of ROTA is important because non-glaucomatous optic neuropathies such as NAION and optic neuritis may have unique signatures of rim pallor. In addition, while certain conditions, such as optic disc drusen and RNFL edema, may compromise conventional OCT analysis in detecting RNFL defects, ROTA can identify these RNFL defects.
Limitations
While ROTA has been shown to be more effective in detecting RNFL defects in different optic neuropathies, it has some limitations, many of which are seen in conventional standards such as OCT and red-free photography. For example, in eyes with dense media opacities or motion artifacts, the image quality of ROTA may be obstructed. Further, the interpretation of RNFL defects using ROTA is primarily subjective, similar to red-free photography. [3] Still, ROTA holds the potential to drastically improve clinical evaluations of optic neuropathies in a very feasible manner.
References
- ↑ Geevarghese A, Wollstein G, Ishikawa H, Schuman JS. Optical coherence tomography and glaucoma. Annu Rev Vis Sci. 2021; 7: 693–726
- ↑ Oddone, F. et al. Macular versus retinal nerve fiber layer parameters for diagnosing manifest glaucoma: a systematic review of diagnostic accuracy studies. Ophthalmology 123. 2016; pp. 939–949
- ↑ 3.00 3.01 3.02 3.03 3.04 3.05 3.06 3.07 3.08 3.09 3.10 Christopher Kai-Shun Leung, A.K.N. Lam, R.N. Weinreb, et al. Diagnostic assessment of glaucoma and non-glaucomatous optic neuropathy via retinal nerve fiber layer optical texture analysis. Nat Biomed Eng. 2022; pp. 593-604.
- ↑ 4.0 4.1 Clarice Kai-Ying Su, Philip Yawen Guo MSc, Poemen Pui Man Chan, et al. Retinal Nerve Fiber Layer Optical Texture Analysis: Detecting Axonal Fiber Bundle Defects in Patients with Ocular Hypertension. Ophthalmology. 2023; pp. 1043-1055
- ↑ Christopher Kai-Shun Leung et al. Retinal Nerve Fiber Layer (RNFL) Optical Texture Analysis (ROTA) for Evaluation of RNFL Abnormalities in Glaucoma. Invest. Ophthalmol. Vis. Sci. 2018;59(9):3497
- ↑ Christopher Kai-Shun Leung, P.Y. Guo, A.K.N. Lam. Retinal Nerve Fiber Layer Optical Texture Analysis: Involvement of the Papillomacular Bundle and Papillofoveal Bundle in Early Glaucoma. Ophthalmology. 2022; pp. 1043-1055